Artificial Intelligence or AI sounds so much like that sci-fi movie but it is actually part of our daily lives these days. Pocket smartphones and online suggestions— it’s all around us. A new player called generative AI has recently taken the conversation to an all-new level.
So, what exactly are traditional AI and generative AI and what is the key difference between AI and generative AI? Well, let’s break it all down for you in simple terms. If you’re a geek interested in this buzz or just curious about the buzz, we’ve got you covered.
Let’s dive into these technologies and see exactly how they are shaping our world and what separates them.
What Is Traditional AI?
The other names for traditional AI are Narrow or Weak AI (Artificial Intelligence). This is a kind of specialised tool designed to do a specific task or set of tasks following pre-programmed rules and algorithms. Unlike the broad, adaptable intelligence we associate with humans, traditional AI sticks to its programming, excelling in limited areas but not beyond.
A possible example could be a customer service chatbot, navigation systems used in autonomous vehicles, or even a chess-playing algorithm.
Key Characteristics of Traditional AI
Programmed Intelligence
The classic AI works according to its programming limits. It does not think on its own; it merely obeys programmed commands given by the creator. For example, a chatbot will be able to answer your questions but can’t go beyond its programmed answers.
Specific Applications
These AI models are made for particular tasks. For instance, a self-driven car is trained to safely navigate the road, but at the same time, it cannot start diagnosing diseases or writing an essay.
Data-Driven Analysis
Traditional AI analyses data to make predictions, which might be used in general forecasting or trend analysis. For instance, a financial AI model would analyse trends in stocks and predict market shifts.
Limited Learning Abilities
The learning capability of narrow AI is directly proportional to the data it is exposed to. It cannot learn anything independently but acts purely on the basis of the data provided by humans in order to function.
Benefits of Traditional AI
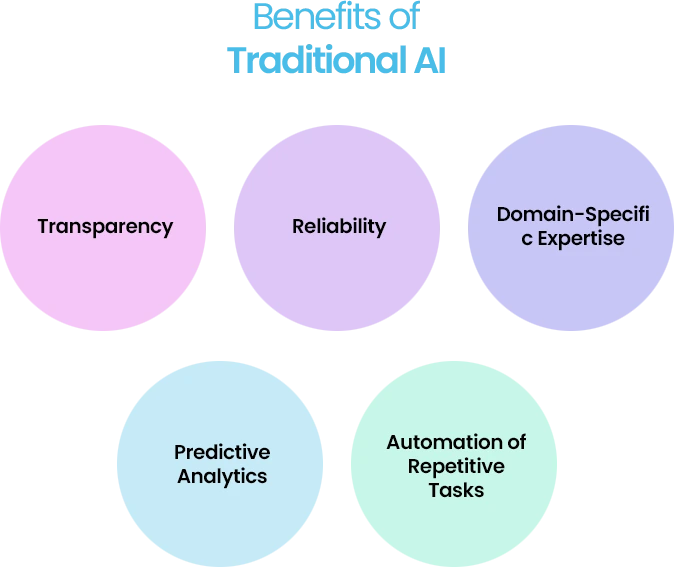
1. Transparency
Classic AI works strictly on well-defined, explicit rules, and hence simple to understand and follow. Because of this transparency, users can follow the decision-making process, which facilitates debugging and improvement.
2. Reliability
With Traditional AI, you would always end up getting the same results. Since it runs on specific rules, it produces predictable outcomes when given the same input. And for any given situation where consistency counts, it’s just great.
3. Domain-Specific Expertise
Traditional AI works very well on specialised domains where it becomes possible to set clear rules in place. For example, in medical diagnosis or finance, there are experts with in-depth knowledge who set clear rules and apply them to solve certain problems through Traditional AI.
4. Predictive Analytics
Traditional AI can sift through tremendous volumes of data and outline trends and patterns to make predictions. This helps businesses make better decisions like forecasting sales or guessing the needs of customers.
5. Automation of Repetitive Tasks
For automating processes like data entry and report generation, traditional AI is ideal. That not only saves time but also reduces the chances of human errors and makes it even more cost-effective.
Limitations of Traditional AI
- Limited Adaptability: The traditional AI is based on a fixed set of rules. If there’s a change in the environment or new patterns emerge, it requires manual updates to keep up – not ideal for fast-changing settings.
- Challenges with Scalability: The traditional AI systems are difficult to scale up. Each new task or situation requires its own set of rules, making it tough to scale up without creating a massive, hard-to-manage rulebook.
- Lack of Generalisation: Traditional AI functions in a narrow framework. It doesn’t have the flexibility to apply its knowledge to new areas outside its programming, limiting its versatility across tasks.
- Limited Learning Capacity: As opposed to more advanced AI systems, traditional AI cannot learn or improve by itself. It depends entirely on the data and rules it’s initially given, which restricts its ability to evolve or get “smarter” over time.
- Lack of Creativity: Traditional AI only develops responses it had present within its data. It cannot create new or innovative solutions; which limits its use for tasks requiring out-of-the-box thinking.
Real-World Examples of Traditional AI
- Google Search Algorithm: Google search depends on classic AI algorithms to deliver relevant results about a certain query, making it easier and faster to access information in a second.
- Voice Assistants (Siri, Alexa): Voice assistants generally use rule-based AI to answer specific queries, whether you’re asking for the weather, setting reminders, or controlling smart home devices.
- Recommendation Engines (Netflix, Amazon): When Netflix suggests a show you might like, or on Amazon that recommends something, that’s basic AI at work. It uses the patterns from your previous choices to make suggestions based on your patterns of interest.
- IBM Watson for Healthcare: IBM’s Watson AI uses basic algorithms to comb through health data. It has since been adopted by doctors to diagnose conditions or provide treatment by matching symptoms to medical knowledge databases.
- Self-Checkout Systems: The self-checkout counters at stores exhibit the traditional AI where it reads the items and goes on to process the payment, thus making the experience of shopping easier because it only does repetitions of fixed routines.
What Is Generative AI?
Generative AI is an incredibly creative digital assistant, which doesn’t respond to simple questions but rather creates new content based on the patterns and data it has been trained on. Imagine it as an AI that can write stories, generate images, compose music, and so on. Generative AI produces new, original content by identifying patterns in enormous volumes of data.
To learn more about the capabilities of large language models and their applications in generative AI, enrol in our course on Generative AI with LLM Certification Course (Large Language Models).
How Does Generative AI Work?
Well, generative AI uses machine learning to analyse and understand large data sets. When you give it a prompt—a few words, an image, or some other instruction—it uses what it’s learned to create something new that fits your request.
These prompts can be text-based, like asking it to write a poem, or may involve other forms, like creating a custom graphic, or even generating a new audio clip.
To dive deeper into the technical aspects of generative AI, consider enrolling in our Generative AI with OpenAI, LlamaIndex, and LangChain Certification course!
Characteristics of Generative AI
Neural Network Generators
Generative AI employs the most advanced types of neural networks: like GANs (Generative Adversarial Networks) and VAEs (Variational Autoencoders), to create fresh, original content.
Wide Range of Applications
This technology isn’t limited to one industry or one type of task. Generative AI can adapt to almost any field, from art and entertainment to finance and healthcare, producing diverse types of content with ease.
Prompt-Based Content Creation
The most striking thing about generative AI is that rather than merrily analysing existing data, it can actually produce new stuff from prompts. By feeding it specific prompts or data, you can get outputs that are entirely unique and directly relevant to your needs.
Benefits of Generative AI
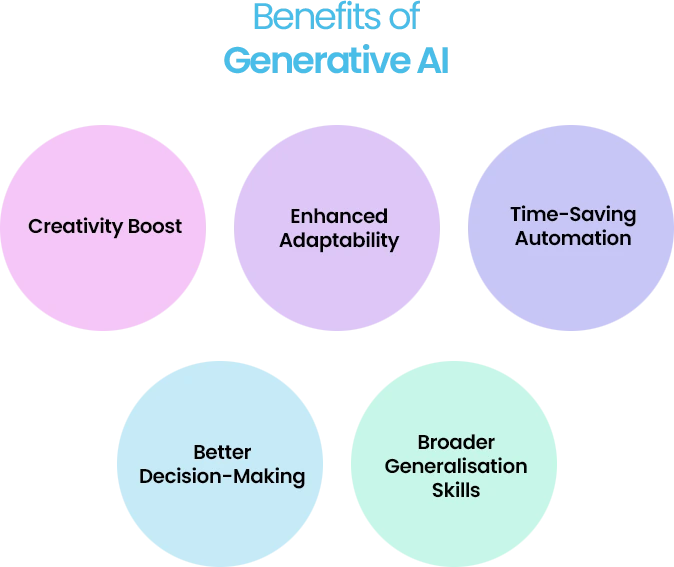
Creativity Boost
Generative AI thrives on data, learning patterns and creating new content from scratch. This makes it ideal for creative tasks like designing images, translating languages, and recommending personalised content. It can inspire fresh ideas on what to produce to keep things innovative.
Enhanced Adaptability
What differentiates the generative AI is that it can learn and adapt to new environments and data, as needed. It does not require frequent updates manually; the more it processes data, the more improvement takes place over time. Its self-learning capabilities keep it relevant and reliable in fast-changing conditions.
Time-Saving Automation
Generative AI can take over mundane tasks; hence, your team has the necessary time to think about what really matters. Automated mundane work enhances productivity and allows workers to engage in more critical tasks.
Better Decision-Making
Generative AI, through the rapid analytics of big datasets, can provide insights to guide wiser decisions. This can come in very handy for businesses to strengthen their strategies and respond immediately to prevailing market changes.
Broader Generalisation Skills
Generative AI has a unique strength in applying its learning across different tasks within the same domain. In other words, it can be applied across many roles, enhancing flexibility and reliability in your processes.
Limitations of Generative AI
- Complexity and Resource Demands: It is difficult to build and train generative AI models. Their good performance requires significant amounts of data as well as a large amount of computing power. So they tend to be expensive and take much time to design, especially for smaller organisations which might not have a big budget.
- Transparency Issues: The complex workings of deep learning models make it very difficult to understand the way they reach their conclusions. Consequently, the lack of transparency here leads to accountability issues; one cannot always answer why a model yields a certain output.
- Risk of Inaccurate Information: Generative AI tends to generate content that sounds plausible but is entirely made up. This is called “hallucination,” and in an attempt to be creative, it would create news or information that could easily be mistaken for facts.
- Outdated Knowledge: Many generative AI models train on outdated information and, by their very nature, lack new updates related to current events. This, of course, limits their effectiveness in answering questions related to current topics or recent occurrences and, thus, may decrease their reliability in fast-moving situations.
Real-World Examples of Generative AI in Use
- Google’s PaLM: Google AI is going to the next level with PaLM. It is a powerful model which is used to develop creative tools like a music generator and a code generator. This technology can be used by musicians while seeking new compositions and programmers can code more efficiently.
- Meta’s LaMDA: Meta AI is employing LaMDA in an effort to make social interactions more exciting by making chatbots feel more natural and conversational. Imagine having a conversation with a bot that actually feels like chatting with a real person—LaMDA is making that possible.
- Microsoft’s Megatron-Turing NLG: Megatron-Turing NLG is being used by Microsoft Research to revolutionise manufacturing. It is a more advanced system for AI, which will assist designers to make products in ways never before possible-undeniably speeding up the development process and driving innovation in product design.
- DALL-E by OpenAI: DALL-E is an image-generation tool which can design unique visual images from certain prompts. Want to see a scene of a dog in a detective outfit or some surreal landscape? DALL-E can bring those ideas to life, making it a favourite for creative industries and beyond.
- AI-Powered Job Search Tools: AI-powered job search is becoming popular. Tools like Resume.io and Jobscan leverage AI to create compelling resumes and cover letters tailored to specific job postings. These tools analyse job descriptions, identify keywords, and suggest relevant skills and experiences to highlight.
Traditional AI vs. Generative AI: Key Differences
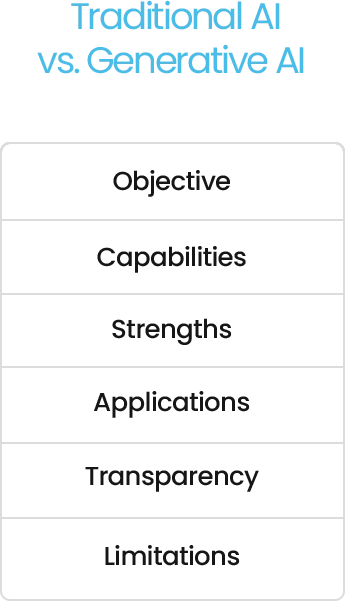
Aspect |
Generative AI |
Traditional AI |
Objective |
Focuses on creating new content, such as text, music, or images, based on input and training data. |
Solves specific problems by analysing data and following predefined rules or patterns. |
Capabilities |
Excels in creativity and innovation. Generates content and ideas, offering novel solutions. |
Specialises in tasks like pattern recognition, prediction, and data-driven problem-solving. |
Strengths |
Flexible, imaginative, and capable of generating original content. |
Transparent, efficient, and reliable for task-specific operations like fraud detection. |
Applications |
Used in content creation, design, entertainment, journalism, and other innovation-driven fields. |
Common in spam filtering, recommendation systems, predictive analytics, and operational tools. |
Transparency |
Complex algorithms can make decision-making harder to interpret. |
Operates with clear rules, making outcomes easier to understand and explain. |
Limitations |
May struggle with precision in data analysis or repetitive task execution. |
Lacks the creativity and adaptability of generative AI. |
Applications of Traditional AI and Generative AI
Application Area |
Generative AI |
Traditional AI |
Creativity and Design |
Digital art, music composition, creative writing, and designing materials or prototypes. |
Game-playing strategies and rule-based problem-solving. |
Healthcare |
Drug discovery and development of personalised treatments. |
Medical diagnosis and patient monitoring systems. |
Technology |
Code generation and innovative solutions for software development. |
Powering self-driving cars and enhancing voice assistants. |
Finance |
Creating predictive models for market trends. |
Fraud detection and financial trading systems. |
Language and Media |
Generating realistic images, videos, and human-like text. |
Machine translation and improving customer experience through chatbots. |
Recommendation Systems |
Building unique suggestions for users, such as custom playlists or designs. |
Personalised product recommendations based on user preferences. |
The Future of AI (Artificial Intelligence)
Traditional AI can be used to complement generative AI and provide emerging solutions. For example, traditional AI can analyse data, while generative AI can use that data to create tailored outputs like personalised content. Countries like Australia, with a strong adoption rate of 68% of businesses already using AI, are leading the way in this technological revolution.
Both AIs are of great importance in contributing to future entities. Traditional AI is great for task-specific operations. Generative AI, on the other hand, fosters innovation and creativity. Together, they open endless opportunities.
The journey of AI is just beginning. With its value projected to increase by over 13x in the next 6 years, AI is reshaping our world. It is acknowledged that we can unlock both of AI’s full potential to overcome challenges and seize opportunities in our fast-evolving digital world.
Staying updated on AI advancements is vital. Courses like the Salesforce AI Associate certification can prepare learners to leverage AI tools effectively and position themselves for success in the future.
Customers and businesses embracing these technologies will find a competitive edge in the future.
Final Thoughts
When it comes to Generative AI and Traditional AI, it’s not about which is better but understanding their strengths. Traditional AI, often called Narrow AI, may seem limited, but it’s unmatched in precision tasks like analysis and predictions. We count on it for its dependability and clarity in specific applications.
Generative AI is something different, operating at new levels of creativity. It can design original content for marketing, e-commerce, and entertainment industries and also adjust to a broad scale of challenges. Its flexibility and innovation make it a key player in today’s AI landscape.
They can be applied in combination to provide solutions for solving problems, predicting trends, and generating unique solutions that neither can achieve alone.
Whatever the case, one thing is clear: AI is here for good, changing the way we live and work.